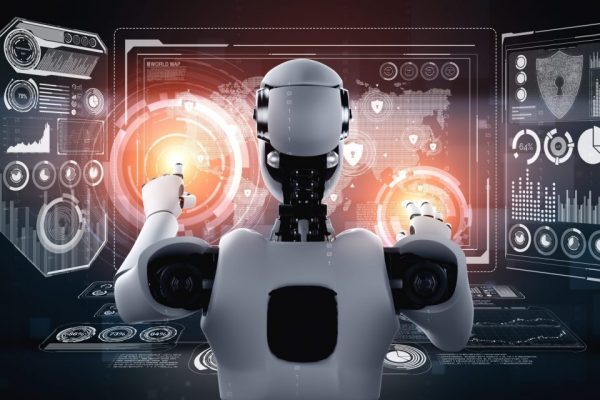
The rise of Artificial Intelligence (AI) has introduced new risks and vulnerabilities in the realm of cyber security. According to Forbes 76% of enterprises have prioritised AI and ML in their IT budgets with Blackberry finding that 82% of decision makers planning to invest in AI driven cybersecurity within the next two years. As AI grows in power, it becomes increasingly susceptible to malicious exploitation, such as data poisoning and manipulation. This necessitates businesses worldwide to be careful when implementing the use of AI and to bolster their existing cyber security measures. In this blog, we will break down what AI, Machine learning and Generative AI are in their simplest form before moving onto five AI risks and vulnerabilities to look out for within the context of cyber security.
What is AI & ML?
Artificial Intelligence (AI):
AI involves training computers to perform tasks that typically require human intelligence. It entails programming computers to think and make decisions akin to humans. For instance, imagine teaching a computer to comprehend and respond to spoken language, enabling you to have conversations with it as you would with a friend. AI can also aid computers in playing games, analysing vast datasets to identify patterns, and even controlling robots to execute tasks.
Machine Learning (ML):
Machine Learning is a facet of AI that focuses on enabling computers to learn and improve from experiences, much like humans do. Instead of providing specific instructions for each task, you provide examples and allow computers to discover patterns independently. For instance, if you expose a computer to numerous pictures of various animals and indicate which ones are cats and which are dogs, it can learn to recognize cats and dogs autonomously. This means that the computer can enhance its performance as it encounters more examples.
There are different types of Machine Learning, including “supervised learning,” where computers learn from labelled examples; “unsupervised learning,” where they identify patterns on their own; and “reinforcement learning,” where they learn through interaction with an environment and receive rewards for positive actions.
Generative AI:
Generative AI takes a step further by granting computers the ability to create new content, such as art, music, or text, based on learned patterns. For instance, if you expose a computer to hundreds of paintings by renowned artists, Generative AI enables it to generate new paintings that resemble the style of those artists, even though they were not created by them. It’s akin to the computer’s way of being imaginative and creative.
Generative AI employs complex algorithms to generate new content, whether it’s producing realistic human faces, composing original music, or even crafting narratives. It’s akin to asking the computer to “imagine” what new creations could look like based on its prior exposure.
In essence, AI aims to make computers intelligent, ML empowers them to learn and improve independently, and Generative AI adds a touch of creativity by enabling computers to generate novel content based on their learning. Whilst implementing AI can be hugely beneficial it can also come with risks.
5 AI risks to look out for
1 – Adversarial Attacks and Manipulation:
Adversarial attacks exploit vulnerabilities in AI models by subtly altering their input data. These alterations, though undetectable to the naked eye, can significantly change a model’s output. As AI technology becomes more widespread, malicious actors will be more motivated to create and launch such attacks. Attackers can create seemingly harmless inputs that trigger malicious actions upon execution. This manipulation can lead to incorrect decisions by AI systems, allowing threats to go unnoticed or even misidentifying safe activities as harmful.
2 – Data Poisoning and Mislearning:
Data poisoning refers to injecting malicious or manipulated data into AI training. In cybersecurity, this can cause AI systems to learn from compromised data, leading to incorrect identification of threats or vulnerabilities. For example, if an AI system is trained on a dataset with altered security logs, it may ignore certain attack patterns, leaving it vulnerable to exploitation.
3 – Biased Outcomes and Discrimination:
AI systems learn from historical data, which may unintentionally include biases found in society. In cybersecurity, this can lead to biased decisions, unfairly targeting or overlooking certain activities or individuals. For instance, if the training data mostly consists of specific types of cyber threats, the AI system can prioritise those and neglect others, resulting in an imbalance in its ability to detect threats.
4 – Human Complacency and Over-Reliance:
The convenience and efficiency offered by AI can lead to human cybersecurity professionals relying too heavily on AI systems. This over-reliance can result in professionals becoming complacent and not thoroughly investigating or validating AI-generated alerts. Attackers can exploit this human tendency, bypassing AI-based defences that security experts may have otherwise identified.
5 – Emergence of AI-Driven Attacks:
The evolution of AI technology brings both benefits and risks. While AI can bolster cybersecurity, cybercriminals can exploit it to create advanced and automated attack methods. Detecting and mitigating these AI-driven attacks with conventional cybersecurity approaches becomes more challenging. Therefore, innovative countermeasures are crucial for effective defence against such threats.